5 Ways your finance team
can benefit from data science
Data science for business intelligence and finance

Today, we share with you five reasons why data science can be a valuable asset for your finance department. As you know, we at Shapelets love to find new innovative solutions to help businesses achieve success in their business intelligence strategies. Get ready to level up your technical skills. But, let’s bring some context first.
Data scientists must have strong technical skills, such as statistics, data architecture, or coding. But they also need to have a good understanding of the business. In fact, data science can help businesses solve problems in different areas, such as optimizing efficiency or process automation. This is why data analytics professionals can support teams and help them perform better, particularly in finance.
Data science has become an essential tool for quickly and easily analyzing data, reducing the need for human input. Financial institutions and departments have long been reliant on data to make sound decisions. As artificial intelligence and machine learning continue to develop, this reliance is only going to increase. Let’s see some of the multiple data science applications in finance:
“What are the five main applications of data science in financial institutions and financial departments?”
Risk Management and Analytics
Risk management is the process of measuring and understanding the frequency of loss, and exploring the potential impacts on a company. There are different types of threats that the company could face, such as from markets, competitors, and credit ratings.
Risk analysis is an important part of data science and business intelligence in finance departments, and is used for making decisions about strategy and efficiency optimization, and security. While traditional structured data can be accommodated in a spreadsheet, more advanced forms of data are not structured and can’t be measured in a continuous manner.
Data science helps make data ingestion easier by monitoring it in real-time and using machine learning to automate tasks and provide advice and alerts when necessary. In the banking industry, for example, machine learning can help identify which factors may lead to a customer’s default.
Real-Time Analytics
Real-time analytics allows us to analyze data as it happens, rather than waiting for it to be collected. This lets for more accurate predictions about future events with data science. In fact, business intelligence and data analysis have relied mainly on structured data for the past few decades.
When analyzing data, the data imported is specific, well-organized, and could be ingested in batches over a long intervals. With the increase in access to social media and online banking and the proliferation of smartphones, data emissions have been growing rapidly. Consequently, it has become more difficult to keep track of and manage this big data.
Today we require the ability to process a continuous flow of unstructured data without getting bogged down. The goal of automating real-time data inflow analysis is to help businesses understand what is happening now and use machine learning and predictive models to have a competitive edge. At its core, real-time analytics can help to personalize the customer experience to an incredibly detailed level.
Consumer Analytics
Understanding your customer is essential to a successful business intelligence strategies. Many financial institutions have put a high priority on customer experience and personalization. Data science can help us gain insights into customer behavior, and it could be beneficial to maybe propose a personalized product based on their preferences.
One example of a predictive analytics technology that is relevant is forecasting which customers are likely to leave the company. Most business leaders know that it is more expensive to acquire new customers than to keep customers. However, if you don’t know why they are leaving in the first place, it can be difficult to keep them. By using a combination of risk analysis, risk management, real-time analysis, triage, and correlation analysis, problems related to customer churn can be easily identified even before they occur.
Fraud Detection
Financial institutions are concerned about fraud, and it is one of their top priorities and even more because of the increase in technology. Traditional fraud detection techniques use a rule-based model that looks for unusual activities. This can often flag actions that have been considered fraudulent or that have violated company policy. The second issue with traditional fraud detection is the increasing amount of data.
For example, let’s take a look at how banks deal with their transaction fraud detection strategies. Digital payment (debit card, electronic payment, credit card) accounts for more than 70% of the total transactions while the consumption of goods and services is developing at the same time. This means that old models can’t keep up with the data flow and are slower, so they need human interaction to prevent fraud. Additionally, because people are already familiar with these models, it’s harder to spot potential fraud using them. By contrast, machine learning algorithms are able to handle a vast amount of data with many variables to find hidden correlations between user behaviors and the likelihood of fraudulent actions, and with a low human error rate.
Algorithmic Trading
Algorithm trading is already more efficient than human traders and does not involve emotions, which makes it an ideal choice for traders. The use of complex mathematical calculations to help advisors and financial companies facilitate the making-decision process to increase profits.
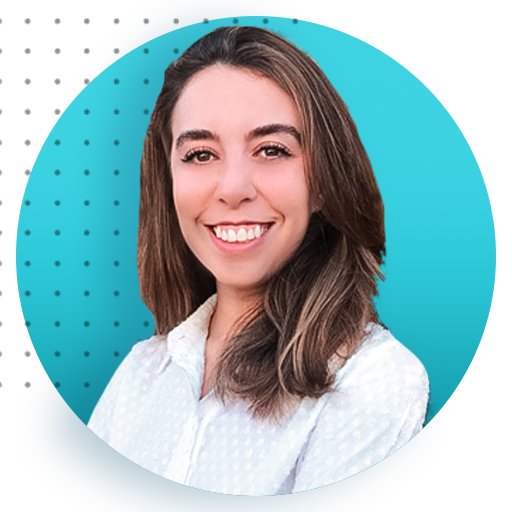
Fátima Ramos
Digital Marketing Specialist