Behind the data scientist
MATÍAS ÁVILA

Welcome back to
Behind the data scientist
Introducing Matía Ávila from IKEA & Lecturer at University of Navarra
Today, we will discuss the different backgrounds of data professionals, the challenges they face, and the skills they use on a daily basis.
The series allows us to explore different topics in the data science community. We will have experts on hand who can provide a comprehensive understanding of the role and experience of a data scientist.
For this first interview, we are joined by Matías Ávila, who is a Data Scientist at IKEA and a Machine Learning Lecturer at the University of Navarra Big Data Science Master Degree.
Watch the interview here below, don’t miss it!
How did you move from Economics to Data Science?
Matías Ávila – When I was doing my undergrad at the University of Navarra while studying Economics, I really enjoyed some lectures such as Econometrics, Time Series, … I was really interested in understanding how some features can explain or even predict behaviors and features values. So, during this time, one of my teachers, suggested me to do my senior undergrad thesis at a time when I had to deal with medical data. So I had to learn Python, and I had a great time gathering data, cleaning it, plotting, modeling… It was then that I discovered Data Science and when I decided to move to the States in order to pursue my career in AI and Big Data. So I started my master’s in Data Analytics at North Caroline State University. So, thanks to this professor, and this senior thesis project is why I moved from Economics to Data Science.
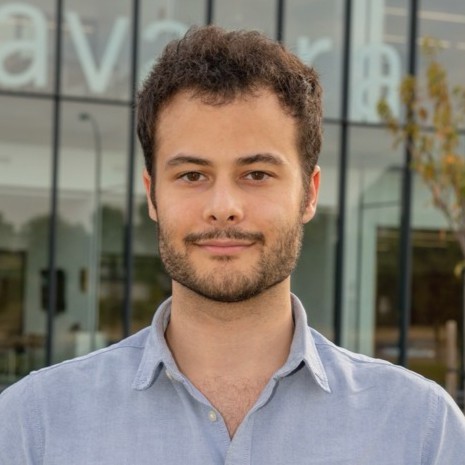
What do you like the most about your job?
M.A. – I always enjoy exploring uncharted patterns within data, that’s always fun. But something I really enjoy is that Data Science is a really eclectic domain. You derive ideas from abroad and a huge range of sources. For example, you have different tools for survival analysis which is quite common in medical trials, models that are brought to the time series domain, algorithms from computer science, or even different metrics such as entropy, which is used in chemistry and physics. So I would say that something I really enjoy about Data Science is that is an area where you interact with very different domains.
What do you value the most in a data analysis tool or a data technology?
M.A. – Something I really value is open-source technologies, because you have a huge community you can contact if you have any problems. Or just if you want to learn how others have solved the very same problem but in a different industry. It’s a great way to understand the tool you are using and get the most out of it.
Who is your role model?
D.R. – Many names come to mind: Raúl Peláez (Ailite), Javier Fernández (Zelus Analytics), Mario Prieto (Atlético de Madrid), Jesús Lagos (Scout Analyst), Sergio Llana (FC Barcelona), Manu Heredia (Be Soccer), Laurie Shaw (Manchester City), Ian Graham (Liverpool), David Sumpter… But I would have to choose only one, I would say the role model that I have closer, Javier M. Buldú, who is a scientist working on football analysis in Football and network analysis group with me.
From your professional experience, what do you find to be the main problems in the connection with the business area?
M.A. –So I would say that there are two main problems. The first would be dealing with expectations. Some people, especially managers, tend to think that Data Science is some kind of magic where you bring a bunch of nerds and blah blah blah they will make a ton of money and fix everything with this algorithm. It doesn’t work like that. First, you have to be sure you have the needed data to solve this problem. Follow up on the solution you’ve implemented. So there are many other things going on behind the scenes.
Another problem I’d say is that usually data scientists tend to lack soft skills. Data scientists have great backgrounds in computer science, mathematics, and engineering, but generally, they struggle when communicating valuable insights or solutions.
So I’d say those are the main problems, dealing with expectations and the lack of soft skills of some data scientists when communicating some ideas to their managers.
Could you please share what is your approach when solving any data analytics-based project?
M.A. –There are different steps you should follow when doing and deploying a machine-learning solution. You have to find a hypothesis, you have to find some metrics in order to track how good or bad is your solutions, you have to train the model, and before that, you have to understand the data. But most people don’t know that. Something I really stress, or something that is really important and most people miss is that you have to get to know the business. Wonder do I know how the warehouse store the goods? Do I really understand what problems truck drivers face when dealing with a shipment? do I know how data is gathered?
If you don’t know or you don’t understand the business, you won’t do your best when analyzing the data, when you are cleaning it, during some imputing values, or even during modeling. So, I’d say that the very first stage that you should focus on is to really get to know the business and then follow the common steps of machine learning.
What advice would you give to maybe your students or to other data scientists?
M.A. –Well, maybe you are from a business background, or maybe from a computer science degree, or you studied medicine. It doesn’t matter. Something you have to do is to take advantage of your knowledge and say, ok, now if I want to turn into a data scientist, what am I missing? If you have studied business, maybe you lack some statistical knowledge. Or even some programming languages.
I strongly recommend you is to learn Python, R, SQL, and Git…but you have to take advantage of the current skills that you have. Let’s say you have studied computer science background, you already know how to program. You just have to focus on honing your skills in statistics and maybe in some business or soft skills.
So what matters is how you use your skills. Let’s say you studied business to become a great data scientist in a bank. If I have studied medicine I’m gonna focus on becoming a great data scientist in the pharma industry. So that’s something I really recommend you, to take advantage of your background. Because that’s what makes you different, also what makes you stand out from the crowd.
Another thing I would strongly recommend is that nowadays, cloud technologies such as Google, Azure, or Amazon. That knowledge is quite important nowadays when working in a big company.
What do you think are the key skills a data scientist need in 2022?
M.A. –I’d say you need three main skills. First, you need some knowledge or skills in computer science, algorithms, also databases. That would be the first one. Then you need some knowledge of statistics and mathematics which is essential in order to deal with some machine learning algorithms. And finally, but not least, you need some soft skills or business knowledge. The combination of these three sets of skills is what makes you a great data scientist.
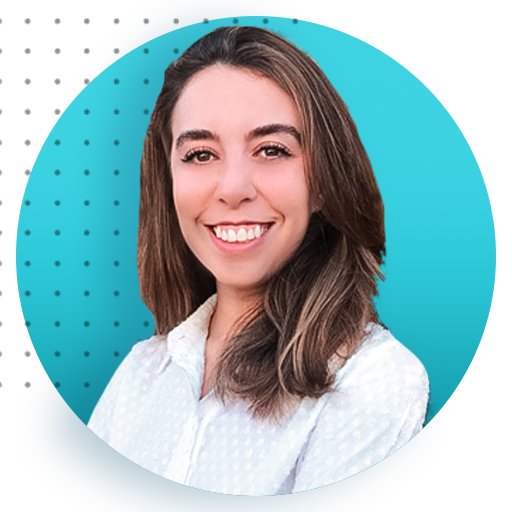
Fátima Ramos
Digital Marketing Specialist
Say hello to our Digital Marketing Specialist! Fátima’s role at Shapelets is to plan and execute digital marketing strategies and content to creatively develop and optimize our business on different platforms. She specializes in SEO, social media and digital content.