Data science roles
in th energy industry
Data science is an area of constant change. In the field of artificial intelligence, deep learning models have recently become very popular for their accuracy

Since the methods of data science are diverse and this is a dynamic field, its potential to change industries sectors, including the energy industry and engineering, is enormous.
The energy industry is also constantly evolving, and more significant inventions and innovations are yet to come. Energy use has always been involved in other industries such as agriculture, manufacturing, transportation and many other industries. These industries tend to consume more power every day.
This is why energy seems to be very demanding in terms of applying new technologies and developing new sources. This rapid development of the energy industry and utilities directly affects social development too. Let’s see a few examples of how data science plays a role in the energy sector:
Failure probability modelling
Failure probability modelling is an important part of the energy industry and it is becoming more popular each day. The active use of the application of failure probability modelling can improve performance, anticipate functional failures, and thus reduce maintenance costs.
The energy companies invest enormous sums in the maintenance and proper functioning of their machines and devices. Unexpected failures in their operations lead to significant financial losses. In addition, the situation has become critical for the people who rely on these companies for energy.
As a result, the reliability and image of the energy provider may be detrimentally affected. The output of a failure probability model application is an important part of a company’s decision-making process. This provides an excellent opportunity to be one step ahead for the company’s management.
Outage detection and prediction
Despite the efforts made by the energy companies, power outages still occur, leaving a considerable number of people without power. In this respect, the power outages are viewed more as a failure of the power grids. However, power failure is a preventive measure due to the automatic operation of the protection system.
In recent years, power system engineers have tended to use static algorithms and models rather than real-time solutions. Many energy and utility companies are now actively upgrading their systems to improve outage detection and prediction. Modern intelligent communication systems for power outages can help on the following:
Fault detection and prediction starts with identifying the right metrics and their threshold. Every outage event should be analyzed to determine the root cause. You can then apply a prediction algorithm to model the potential for future outages. The application of the Smart Outage Ecosystem allows providing accurate real-time outage status to improve general customer experience and satisfaction.
Dynamic energy management
Dynamic power management systems belong to the innovative approach to load management. This type of management covers all of the traditional energy management principles for demand, distributed sources, and demand-side management, as well as modern energy challenges such as savings, temporary load, and demand reduction. Therefore, advanced energy management systems have been able to combine smart devices, distributed resources, and advanced control and communication technologies to improve energy efficiency.
Big data analytics plays a leading role here as it supports dynamic management systems in smart grids. This helps optimize energy flow between providers and consumers. The effectiveness of the energy management system, in turn, depends on load forecasting and renewable sources.
The dynamic energy management component typically includes intelligent energy terminals, intelligently distributed energy sources, advanced control systems, and integrated communication architecture.
Dynamic energy management systems process huge amounts of data obtained through practical methods and solutions. Applying big data analysis to this data can help you estimate performance and provide smart recommendations for energy management.
Optimizing Plant Performance
Any potential outage or delay in power supply, unplanned downtime or complication leads to inefficiency. By monitoring performance and assets, this inefficiency can be prevented or at least controlled. Real-time asset health data, supply and demand analytics help improve asset performance.
Data-driven and business intelligence-driven tools and software are used to monitor conditions, costs, and performance, and to identify scoring methods and areas of critical priority. Thus, they enhance the reliability, capacity, and availability of the assets, while minimizing costs. The more data you have, the more you can do to better manage your assets.
Preventive equipment maintenance
Preventative equipment maintenance is based on monitoring current equipment health and performance levels under normal operating conditions. This monitoring is used to predict possible failure based on specific metrics. To achieve the maximum return on investment and keep complex machinery and equipment operating at their peak efficiency, power distribution and utility companies have been employing preventive asset maintenance for decades.
Smart data solutions, sensors and trackers are used to collect certain indicators, process and analyze data. Based on the output, the intelligent systems warn of the power outage, poor functioning of the mechanisms and prompt people to make correct and immediate decisions.
Smart Grid security and theft detection
Energy theft can be considered one of the most expensive types of theft. Therefore, energy companies take great precautions to prevent it.
Moreover, energy theft in smart grids often occurs via a direct tap in the distribution cable. To predict and prevent energy theft and consequent loss of money, large energy companies and corporations monitor the flow of energy to react immediately to some suspicious matters. With this purpose, business owners tend to switch to advanced metering infrastructure, capable of reporting energy use cases and remote control.
Smart grid security solutions are very popular. These solutions can be behaviour-based, so they constantly monitor user behaviour to detect hackers and reveal their intended activities.
Demand response management
Demand response management is a very popular way to save energy and be efficient. The key to successful energy management lies in the balance between supply and demand. High and low demand rates cause a lot of problems and costs for both energy providers and consumers.
In addition, demand response is an effective strategy over time. Specific real-time management applications and solutions enable monitoring of energy consumption metrics, the definition of activity selection and adjustment of energy flow to the current demand rate. Moreover, there are response management programs that encourage consumers to use energy at a specific time and save money. This means that consumers will have a chance to switch to a better pricing program, while providers will be able to achieve the desired balance in energy provision.
Improving Operational Efficiency
Efficiency itself requires completing certain tasks in less time than previously. The fast pace of modern life and everyday affairs makes people strive for efficiency in everything. The energy industry and utilities use smart data applications and software to detect optimization-worthy problems, operations, and features.
Real-time monitoring provides data regarding the time, activity rate, and status of certain processes. The data is processed in combination with external factors to determine the average efficiency. Data science here is used to model different situations and predict possible efficiency rates in different situations.
Improving Customer Experience
There are two priority dimensions of business for energy and utility companies that are directly related to generic brand reputation. These are two important aspects of running a successful business. They work hand-in-hand to create a positive customer experience. The rapid development of smart technologies and the increasing popularity of smart homes provide new opportunities for users. Customers become more knowledgeable about the best company or service to buy from. Thus, the demand for high-quality services continues to increase. All companies make every effort to satisfy customers’ needs and desires.
First, multiple communication channels should be applied for this purpose. Omni channels provide our company with valuable insights that can be used to improve processing. With accurate analytics, companies can effectively reveal information about their customers’ demographics, behaviours, and emotions. As a result, they can provide personalized recommendations, suggestions, and services that are specific to your needs.
Energy and utilities companies are under constant pressure to provide high-quality services 24 hours a day, 7 days a week, at affordable prices, without delays or obstacles. People rely on energy sources for their daily transactions and work.
Due to the rapid development and improvement of technology, the energy industry faces new opportunities and new challenges every day. Machine learning algorithms, analytical models and big data solutions help companies manage and use their resources effectively, control energy flows, regulate the grid, optimize work and avoid mistakes that can cost a lot.
The use of real-time predictive analytics and data science solutions requires significant investment, a willingness to take on challenges, and learn and introduce new complex processes. However, as we know now, there are many benefits of data science applications in the areas of energy and utilities. It’s our time to change and improve things.
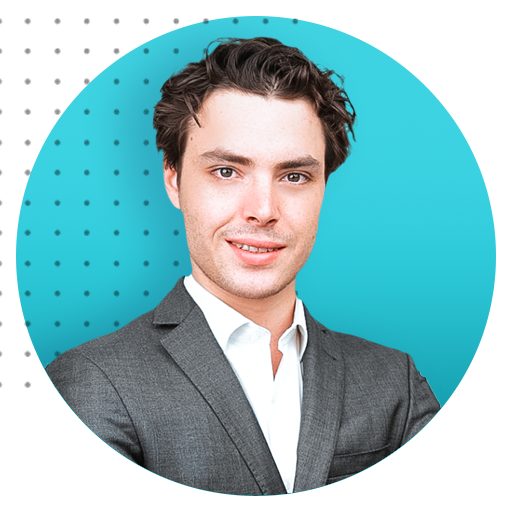
Daniel Ramírez
Engineer
Daniel is a Data Engineer for Shapelets. He is an integral part of the back-end development team, where we develop a high-quality platform ensuring the best product design with valuable functionalities for data scientists. Daniel supports the team with his diverse background in Software Engineering.