DATA SCIENTIST:
The specialization of the
hottest 2020 job
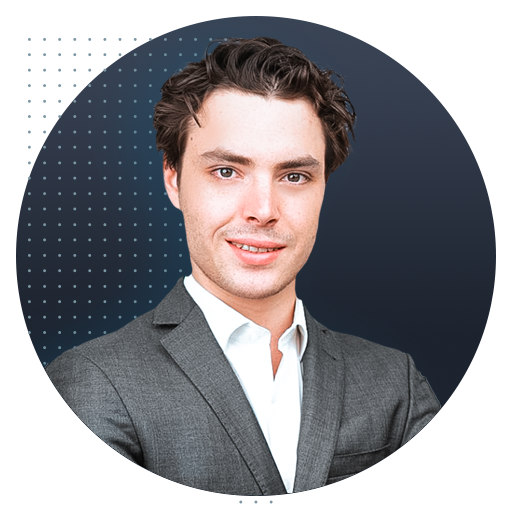

Data Science was considered the hottest job of the century in an article in the Harvard Review in October 2012.
June 2023 | 8 Minutes
Content list
Content list
Intro
Data Scientist: The Hottest Job of the 21st Century
As a nearly recent position, Data Science was considered the hottest job of the century in an article in the Harvard Review in October 2012. They start the article by explaining the history and the need for big data analysis thanks to coding skills. They took the example of “Reid Hoffman, LinkedIn’s cofounder and CEO at the time (now its executive chairman)” who predicted that big data analysis would be useful for presenting to users the name of people they hadn’t met yet on Linkedin, which achieved a click-through rate 30% higher than other prompts.
This was a good example of a new actor in the workforce: “Data Scientist: It’s a high-ranking professional with the training and curiosity to make discoveries in the world of big data”. At that time their role was to focus on technology tools such as Hadoop, cloud computing, and Data visualization. In the article, we learn that Data Scientists at that time realized they were facing technical limitations and a lack of tools, making their work more tedious.
hbr.org
Twenty years after this article, we can ourselves the question, is Data Scientist still the sexiest job of the century and why? Looking at the Bureau of Census from 2021, we learn that the job outlook for 2021-31 was 36%, which was much faster than the average. With increasing but low production of skilled workers, Data Scientists are still highly valuable for a company. To lower the gap between the demand and the offer, new companies have emerged offering low or no-code platforms to help empower workers with a new set of skills. That’s where we are seeing a new emergence of workers called Citizen Data Scientists.
At the same time, Data Scientist has started to diversify itself into much more specialized individuals that will fulfill the needs of the company. There are 9 specializations of Data Scientists that have been listed by Upgrade which are, Data Mining and Statistical Analysis, Business Intelligence & Strategy-Making, Data Engineering and Data Warehousing, Data visualization, Database Management, and Data Architecture, Operations-Related Data Analytics, Machine Learning and Cognitive Specialist, Market Data Analytics, and Cybersecurity Data Analysis.
In this article, we will see the exploration of a paradigm shift in the Data science workforce from the use of new tools to upgrade the skills of analysts to the specialization of Data Scientists.
Data Scientist Specialization
1 /Data Mining and Statistical Analysis
Data mining involves scrutinizing extensive data sets to identify patterns and connections that can aid in data analysis, ultimately assisting in the resolution of business issues. It enables predictive models for exploratory data analysis to find patterns and trends in data. On the other hand, working with data is made easier throughout the entire process of statistical analysis. It makes it possible to strategically infer many facts from the data, which improves final outcomes.
The market for data mining is expanding quickly and has a broad application. Numerous real-world examples are provided, including the forecasting of loan payments, the placement of goods in supermarkets, customer retention, attrition, etc. Data mining involves six primary tasks, including classification, estimation, prediction, and clustering. Data mining and statistical analysis are both methods of learning from data, with the objective of discovering and identifying structures within the data to transform it into useful information.
# 1
Data SPECIALIZATION
2 / Business Intelligence & Strategy-Making
Business Intelligence (BI) is a branch of data science that deals with turning data into insights to help businesses reach their full potential. BI involves accurately and easily managing back-end data sources, providing customized analytics solutions for business-critical issues, and identifying and predicting market competition. By using BI software to mine complex datasets and analyze competitors’ business trends, BI can discover areas of revenue loss and areas where companies need to improve. Business Intelligence (BI) plays a critical role in today’s business world. It allows organizations to gain a comprehensive understanding of their performance and their competitors’ strategies.
With this knowledge, businesses can make informed decisions backed by scientific data, leading to effective strategies. Some of the tools used in BI are Microsoft Power BI, Qlik, Looker, and SAP. Job roles in BI include BI Engineer, Data Strategist, BI Analyst, and BI Developer.
# 2
Data SPECIALIZATION
3 / Data Engineering and Data Warehousing
Data engineering is a specialization of data science that involves converting data into a meaningful format for intense analysis. Data engineers bring together data from various sources and organize it using custom queries to uncover useful insights for making informed decisions.
This specialization entails overseeing the collection, organization, tone, storage, and retrieval of data for other data analysts and data science professionals. Data engineers optimize the data, allowing businesses to reach better usability from the data. The advantages of data engineering include agility, faster and more accurate business decision-making, and better revenue generation as organizations can predict the future and make adjustments accordingly. Data engineers utilize various tools such as Amazon Redshift, Big Query, Tableau, Looker, etc. Job roles in data engineering include BI Engineer, Data Strategist, BI Analyst, and BI Developer. [5][6]
# 3
Data SPECIALIZATION
4 / Data visualization
Data visualization is a specialized domain of data science that involves representing data and information into a graphical representation. Data visualization employs various tools such as graphs, tables, charts, plots, infographics, and maps to help viewers better understand trends, competition, outliers, growth rates, data patterns, and situations.
The primary objective is to simplify the identification of patterns, trends, and outliers in extensive data sets. Business analysts and data scientists specialize in presenting data in visually appealing forms. Visualizing data is crucial in most professions, as it allows teachers to showcase students’ test scores, businesses to evaluate their achievements, and researchers to present their discoveries. Typically, data visualization serves three main purposes: verifying and refining data, exploring and discovering insights, and communicating results to stakeholders in the industry.Data visualization tools include Tableau, Looker, Microsoft Power BI, etc.
# 4
Data SPECIALIZATION
5 / Database Management and Data Architecture
Database management is a specialized domain of data science that involves organizing information and data in a centralized data architecture aligned with industry standards. It minimizes the scope of errors that infiltrate throughout the decision-making process across the organization. The database management system allows organizations to work with larger volume data as it gets stored in one place. There are many tools available for managing databases, including MySQL, Oracle RDBMS, Salesforce, and DevOps. Effective data management involves securely and efficiently collecting, storing, and using data at a reasonable cost. The Data Management function of an organization is responsible for overseeing the acquisition, storage, quality, governance, and integrity of enterprise data.
This function also develops and enforces all data-related policies within the organization. Database management is important for complex data transactions supporting applications, services, or groups of services.
# 5
Data SPECIALIZATION
6 / Operations-Related Data Analytics
Operational-related data analytics is a data science specialization that uses tools and data provided by other employees and members of the organization to find possibilities of improvement within the various operations of the business, such as logistics, technology, human resources, and financials. It allows businesses to streamline their tasks better and provide better operability over their tasks on a real-time basis. The businesses are more reliant on data, so the Operational Analytics streamlines the data directly from the data warehouse to the relevant tools or CRMs that the team uses, such as Salesforce and more. This specialization does not require high technical skills and is suitable for those passionate about problem-solving.
Data analytics plays an essential role in increasing the revenue of the business, responding immediately to developing industry trends, enhancing operational efficacy, gaining a competitive edge over rivals, and identifying website visitors. Data analytics is recognized to support a broad array of business uses.
# 6
Data SPECIALIZATION
7 / Machine Learning and Cognitive Specialist
Machine learning and cognitive algorithm development are two of the top-rated specializations of data science. These specializations involve developing algorithms and Artificial Intelligence (AI) based solutions. Machine learning algorithms can be used to train models, build pipelines of data, A/B testing, convenient data sources, and benchmarking base-systems. Many companies are using AI-powered algorithms to improve their efficiency and predictability. These algorithms are trained using datasets to help machines learn cognitive responses and decision-making skills. To build these algorithms, companies hire machine learning professionals who use industry-standard tools and statistical techniques.
By using these algorithms, companies can reduce repetitive tasks that were previously done by humans, which can lead to increased turnover. This field of specialization is a complex domain of data mining and statistical analysis.
# 7
Data SPECIALIZATION
8 / Market Data Analytics
Marketing data analytics is a role that comes with different verticals of expertise on different levels for a company. Marketing data analysts play a crucial role in measuring, analyzing, and managing the effectiveness of marketing strategies to optimize return on investment. By understanding market trends and customer needs, they can help reduce wasted web marketing expenses and provide insights into customer preferences and trends.
They utilize various tools and techniques such as paid search marketing, marketing software products, and search engine optimization to leverage data for analysis. This allows businesses to identify new opportunities and customers. This role uses external data sources to improve marketing performance and optimize return on investment.
# 8
Data SPECIALIZATION
9 / Cybersecurity Data Analysis.
Cybersecurity data analyst is a data science specialization that combines cybersecurity and data analytics. The role involves analyzing data in the context of cybersecurity. In the field of cybersecurity, data analysis plays a crucial role in detecting bugs and vulnerabilities, predicting future attacks, and improving data security and privacy within organizations. To achieve this, companies hire cybersecurity data analysts who design and implement algorithms using large datasets obtained from various security tools. The analysts are responsible for safeguarding the organization’s resources and plans against both external and internal threats and crimes. As organizations are data-driven, they possess a significant amount of sensitive data that needs protection.
Cybersecurity data analysis helps to prevent threats, predict potential attacks, and provide better protection measures to safeguard against them.
# 9
Data SPECIALIZATION
10 / Data Scientist Citizen
A citizen data scientist is someone who uses predictive or prescriptive analytics to create models, but their main job is not in the field of statistics and analytics. They can help improve a company’s analytics maturity and overall business value, but often their abilities are not fully utilized. To support citizen data science, leaders in data and analytics need to provide a complete ecosystem that includes people, tools, data, and processes. Complementary roles such as business translators, developers, data engineers, and machine learning architects together can support citizen data scientists to fill in the skill gaps that they lack.
The idea of citizen data scientists is having a meaningful influence on the market because it enables organizations to utilize data science without needing to employ a whole team of data scientists. This approach also makes data science more accessible to a broader range of individuals within an organization, which helps democratize it.
Why Data Scientist citizen are important for the business.
Citizen data scientists are important to businesses because they can fill the gap of the ongoing shortage of skilled data scientists. Data scientists are expensive to hire, and they are hard to find due to their specialized technical skills. Organizations can achieve cost savings, improve their analytics efficiency, and make better use of their data resources by utilizing citizen data scientists. They can help organizations predict how customers will respond to marketing campaigns and promotions, identify potential new revenue opportunities based on business trends, optimize supply chain management, customer service, and other operations, analyze financial and reputational risks to avoid possible business problems, develop stronger business plans and strategies, and gain competitive advantages over business rivals. Currently, the field of data science is advancing rapidly. They can boost an organization’s business value and analytics maturity.
Are the Data Citizens threatening the role of Data scientists?
To answer your questions, expert data scientists would not be threatened by the creation of this new type of worker for various reasons.
As stated previously, citizen data scientists are not here to replace expert data scientists (nor would they be able because of the lack of statistical knowledge), However, they are here to complete them. In the same way as the industrial revolution allowed the creation of more specialized positions, the appearance of those new tools will allow analysts in the company to handle the boring part of the data scientist jobs and help the expert to focus on their technical knowledge.
For example, the citizens would focus on the data handling part whereas the data scientist (depending on their specialty would handle the most technical part).
# 10
Data SPECIALIZATION
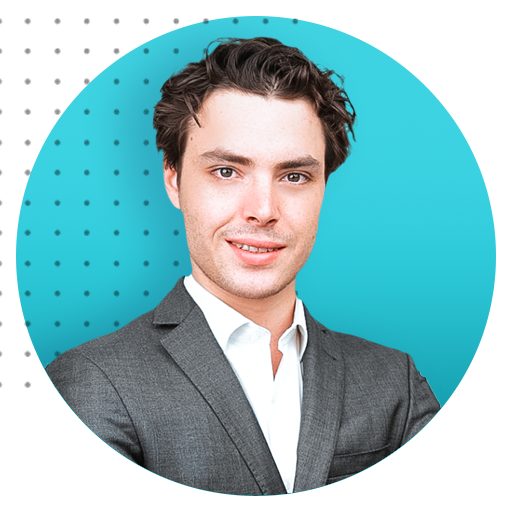
Clément Mercier
Data Scientist Intern
Clément Mercier originally received his Bachelor’s Degree in Finance from Hult International Business school in Boston and is currently finishing his Master’s Degree in Big Data at IE school of Technology.
Clément has good international experience working with startups and big corporations such as the Zinneken’s Group, MediateTech in Boston, and Nestlé in Switzerland.
References
Links
[1] https://www.techtarget.com/searchbusinessanalytics/definition/data-mining
[2] https://www.simplilearn.com/data-mining-vs-statistics-article
[3] https://www.talend.com/resources/data-science-vs-business-intelligence/
[4] https://www.dataversity.net/data-science-vs-business-intelligence/
[5] https://blog.ubiminds.com/en-us/data-scientist-vs-data-analyst-vs-data-engineer-vs-dba/
[6] https://www.upgrad.com/blog/different-specializations-of-data-science/
[7] https://www.techtarget.com/searchbusinessanalytics/definition/data-visualization
[8] https://www.mastersindatascience.org/learning/what-is-data-visualization/
[9] https://www.oracle.com/in/database/what-is-data-management/
[10] https://www.dataversity.net/data-management-vs-data-science/
[11] https://www.techtarget.com/searchbusinessanalytics/definition/data-mining
[12] https://www.techdemand.io/insights/data/analytics/what-is-data-analytics-benefits-challenges-and-use-cases/https://www.simplilearn.com/data-mining-vs-statistics-article
[13] https://csweb.rice.edu/academics/graduate-programs/online-mds/blog/data-science-vs-ai-and-mlhttps://www.talend.com/resources/data-science-vs-business-intelligence/
[14] https://www.dataversity.net/data-science-vs-business-intelligence/
[15] https://www.slingshotapp.io/blog/marketing-data-analysthttps://blog.ubiminds.com/en-us/data-scientist-vs-data-analyst-vs-data-engineer-vs-dba/
[16] https://www.marketingevolution.com/marketing-essentials/marketing-analyticshttps://www.upgrad.com/blog/different-specializations-of-data-science/
[17] https://resources.infosecinstitute.com/topic/data-analytics-in-cybersecurity/https://resources.infosecinstitute.com/topic/data-analytics-in-cybersecurity/https://www.techtarget.com/searchbusinessanalytics/definition/data-visualization
[18] https://www.simplilearn.com/citizen-data-scientists-articlehttps://www.mastersindatascience.org/learning/what-is-data-visualization/
[19] https://www.gartner.com/smarterwithgartner/how-to-use-citizen-data-scientists-to-maximize-your-da-strategyhttps://www.oracle.com/in/database/what-is-data-management/
[20] https://www.simplilearn.com/citizen-data-scientists-articlehttps://www.dataversity.net/data-management-vs-data-science/