Data Science
Trends 2023
As we approach 2023, it’s important to stay up-to-date on the latest data science trends and developments in these areas.

Data science and analytics trends are rapidly evolving fields that have the power to transform the way businesses operate and make data-driven decisions.
But, do you know all of them?
Read further and be ready!
According to a recent article, we can expect 7 key trends to shape the data science and analytics landscape in the coming year. These trends include the rise of self-service and data automation tools, the increasing importance of data ethics and data governance, the growing role of artificial intelligence (AI) and machine learning (ML), the emergence of hybrid cloud architectures, and the importance of real-time data processing. In addition to these trends, there is also an increasing focus on time series databases and data ingestion.
Time series data, which refers to data that is recorded at regular intervals over some time, is becoming increasingly important as more businesses (i.e. in the IIoT sector) look to track and analyze trends and patterns over time. Similarly, data ingestion, or the process of collecting and importing data from various sources, is becoming more critical as businesses seek to integrate data from a wide range of sources and platforms.
The data trends
These trends have the potential to greatly impact the way businesses collect, process, and analyze data, and organizations need to stay informed and adapt to these changes to remain competitive in 2023. Let’s review each of them!
1. Data ingestion
Ingestion of data has evolved into a critical component of the AI and Data Science workflow. Data must first be cleaned, transformed, and loaded into an analysis system before it can be analyzed. This process can be time-consuming and resource-intensive, and data professionals require specialized skills, so businesses should invest in them.
In the field of AI and Data Science, a variety of tools and technologies are used for data ingestion. Data can be ingested from a variety of sources, including social media, IoT devices, and weblogs, using these tools.
The growth of AI and Data Science has also led to an increase in the use of cloud-based platforms for data storage and analysis. These platforms, such as Amazon Web Services (AWS) and Microsoft Azure, enable businesses to store and analyze massive amounts of data without requiring costly on-premises infrastructure. This has made AI and Data Science more accessible to organizations of all sizes, resulting in even more growth in the field.
Overall, the increasing amount of data generated and the need for professionals who can analyze and extract value from this data have driven the adoption and expansion of AI and Data Science. In this process, efficient data ingestion is critical, and the use of the right tools and technologies, as well as best practices, has helped to ensure the accuracy and integrity of the data being collected. With the continued growth of AI and Data Science, the optimization of data ingestion will most likely remain a key focus for businesses and organizations.
2. The rise of automation and self-service tools
Because data volumes continue to grow and the demand for faster and more accurate data analysis grows every day, data automation tools and self-service tools are becoming increasingly important in the field of data science and analytics.
These tools allow users to access and analyze data with no need for specialized technical skills, making it easier for businesses to leverage data insights to inform decision-making and drive growth. Data science automation tools can also help reduce the time and resources required for data processing and analysis, freeing up data professionals to focus on higher-value tasks.
3. Time series database management systems
The global time-series database software market is expected to see significant growth in the coming years, according to a recent MarketWatch research report.
Time series data, which refers to data recorded at regular intervals over some time, is becoming more popular in industries such as finance, healthcare, and manufacturing. The increasing adoption of the Internet of Things (IoT), as more devices are connected to the Internet and generate large amounts of IoT data that require efficient storage, management, and analysis, is one factor driving this growth. Time-series databases, which are specifically designed to handle large amounts of time-series data, are well suited to meet this requirement.
Timeline DBMSs are specialized databases that can rapidly ingest, manipulate, and aggregate IoT data based on its position in a time series. They are especially useful for IoT and financial systems, where the ability to process and analyze large amounts of time-stamped data in a timely manner is critical. This means that time series DBMSs can store detailed data for a set period of time and then generate aggregations based on that data over a longer time frame, as well as compare and analyze data from multiple time streams.
The growth of IoT data sources has fueled interest in time series DBMSs, and major cloud providers such as Microsoft and Amazon Web Services have begun to provide them. Overall, the market for time-series database software appears to be poised for significant growth in 2023 and beyond. Businesses in a variety of industries will likely turn to time-series databases to meet their needs as IoT, AI, and machine learning become more widely adopted, as well as the growing need for efficient data management and analysis.
4. The increasing importance of data ethics and governance
As companies place a greater emphasis on data science ethics and governance, the value of data remains a strategic asset. This trend is being driven by the need to ensure the integrity and security of data, as well as to address privacy and data use concerns. Establishing policies and procedures to ensure the ethical collection, storage, and use of data, as well as implementing security measures to protect sensitive information, are all part of data ethics and governance practices.
5. The growing role of artificial intelligence and machine learning
Businesses that want to gain a competitive advantage through data-driven decision-making are increasingly turning to artificial intelligence (AI) and machine learning. These technologies enable the automation of data analysis tasks, allowing businesses to process and analyze large amounts of data quickly and accurately. AI and machine learning technology adoption is expected to increase further in 2023, with businesses of all sizes looking to leverage these technologies to improve efficiency, drive innovation, and make more informed decisions.
6. The emergence of hybrid cloud architectures:
In 2023, hybrid cloud architectures are expected to become more usual, combining the scalability and flexibility of cloud-based solutions with the performance and security of on-premises systems. The need for businesses to take advantage of the benefits of both on-premises and cloud-based solutions is driving this trend. Hybrid cloud architectures enable businesses to store and process data in the most efficient way possible, allowing them to scale up or down as needed and access data from any location with an internet connection.
7. The importance of real-time data processing:
The proliferation of connected devices, as well as the rise of the Internet of Things (IoT), are generating massive amounts of data that must be analyzed in real-time in order for businesses to make timely and informed decisions.
This trend toward real-time data processing is expected to continue in 2023, with companies looking to harness the power of real-time data to improve efficiency, drive innovation, and make better-informed decisions. Businesses with real-time data processing capabilities can analyze data as it is generated, allowing them to respond to changing conditions and opportunities in real-time.
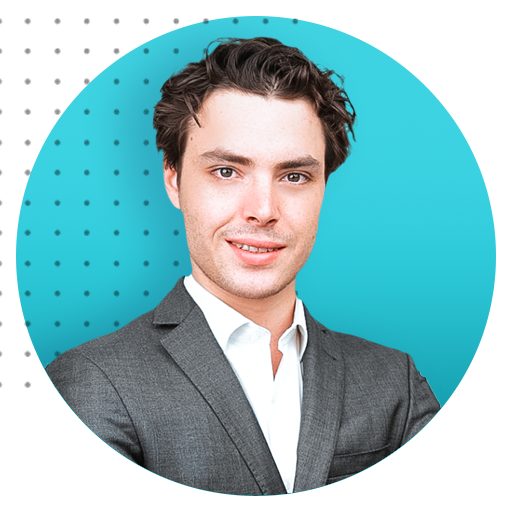
Clément Mercier
Data Scientist Intern
Clément Mercier originally received his Bachelor’s Degree in Finance from Hult International Business school in Boston and is currently finishing his Master’s Degree in Big Data at IE school of Technology.
Clément has good international experience working with startups and big corporations such as the Zinneken’s Group, MediateTech in Boston, and Nestlé in Switzerland.